[Workshop] Causal Inference Workshop
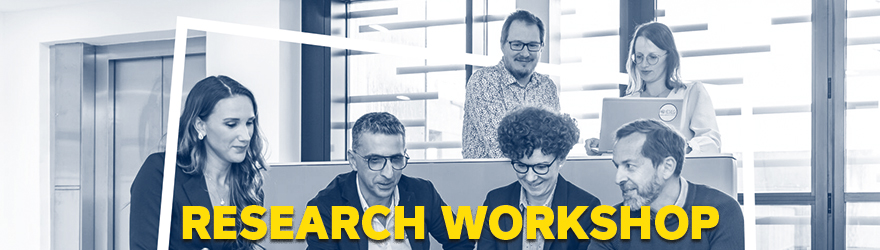
Date and Location – May 20th, 2025 on IÉSEG Lille Campus
9h30am – 5pm
—————————————–
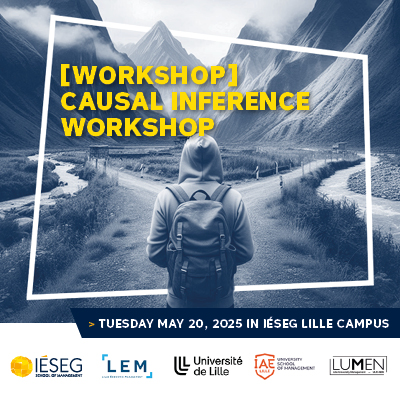
Many research questions in the social sciences aim to establish a causal relationship between a particular element and its expected effects. In recent years, the quality of empirical studies designed to address these questions has significantly improved, particularly through more systematic use of identification strategies previously developed in economics. These strategies stem from economists’ growing awareness of the limitations of certain methods and were recognized with the Nobel Prize in Economics in 20211. Consequently, this “credibility revolution” in causal inference has naturally led to the recent and rapid adoption of the most sophisticated identification techniques, particularly in the field of corporate finance2.
workshop topic
The 2025 workshop will focus on the methods of selection on observables. It will cover the theoretical concept of potential outcomes, Directed Acyclic Graphs (DAGs), regression adjustment, non-parametric matching methods to balance treatment and control groups, semiparametric methods that combine parametric and non-parametric approaches for more robust estimation.
Targeted audience
The workshop is intended for academic researchers interested in empirical work, primarily in causal inference. Although the empirical framework presented is mainly based on examples from economics, finance, and accounting, the fields of application are broad and encompass all areas of management and social sciences (marketing, strategy, human resources, organization, political science, etc.).
Practicalities and Registration
- The day will be moderated by faculty members from IAE Lille and IÉSEG (accounting and finance departments). Registration is free, but compulsory before April 20, 2025.
- The workshop will take place in person at the IÉSEG Lille campus on Tuesday, May 20, 2025. After registering, further details will be provided.
Organizing committee
Jean-Gabriel Cousin (Univ. Lille IAE France – LUMEN), Hicham Daher (IÉSEG – LEM), Marion Dupire (IÉSEG – LEM), Jean-Yves Filbien (Univ. Lille, IAE France – LUMEN), Maia Gejadze (IÉSEG – LEM), Gael Imad’Eddine (Univ. Lille, IAE France – LUMEN), Paolo Mazza (IÉSEG – LEM), Romain Oberson (IÉSEG – LEM), Francesco Roccazzella (IÉSEG- LEM).
program
- 9h00 – 9h30: welcome
- 9h30 – 10h30: introduction and theoretical framework based on [10], [4], [5] and [6]
- 10h30 – 11h30: regression adjustment based on [2] and [12]
- 11h30 – 12h30: matching techniques based on [1], [7] and [10]
- 12h30 – 14h00: lunch
- 14h – 15h: empirical illustration based on [8] and [9]
- 15h – 16h30: group work
- 16h30 – 17h00: open discussion time
References
- Abadie, A., & Imbens, G.W. (2006). Large sample properties of matching estimators for average treatment effects. Econometrica, 74(1), pp. 235-267
- Angrist, J.D., & Pischke, J.S. (2009). Mostly Harmless Econometrics: An Empiricist’s Companion. Princeton University Press
- Bowen, D., Frésard, L. & Taillard, J. (2016) What’s Your Identification Strategy? Innovation in Corporate Finance Research. Management Science 63(8):2529-2548.
- Cunningham (2021). Causal inference: The mixtape. Yale University Press.https://mixtape.scunning.com
- Holland, P.W. (1986). Statistics and causal inference. Journal of the American Statistical Association. Vol. 81, pp. 945-960.
- Huntington-Klein, N. (2022). The effect: An introduction to research design and causality. Chapman and Hall/CRC. https://theeffectbook.net/
- Imbens, G.W. (2004). Nonparametric estimation of average treatment effects under exogeneity: A review. The Review of Economics and Statistics. 86(1), pp. 4-29.
- Imbens, G.W., & Xu, Y. (2024). LaLonde (1986) after Nearly Four Decades: Lessons Learned. Working paper. Available at SSRN https://ssrn.com/abstract=4849285
- LaLonde, R.J. (1986). Evaluating the econometric evaluations of training programs with experimental data. The American Economic Review. 76(4), pp 604-620.
- Rosenbaum, P.R., & Rubin, D.B. (1983). The central role of the propensity score in observational studies for causal effects. Biometrika. 70(1), pp. 41-55.
- Rubin, D.B. (1974). Estimating causal effects of treatments in randomized and nonrandomized studies. Journal of Educational Psychology, 66(5), pp. 688-701.
- Sloczynski, T. (2022). Interpreting OLS Estimands when treatment effects are heterogeneous: smaller groups get larger weights. The Review of Economics and Statistics, 104(3), pp. 501-509.
[1] David Card, Joshua Angrist et Guido Imbens “for their methodological contributions to the analysis of causal relationships” The Sveriges Riksbank Prize in Economic Sciences in Memory of Alfred Nobel 2021 – NobelPrize.org 2 The proportion of academic articles using modern identification strategies was close to zero until the late 1980s, then steadily increased to over 50% by 2012 (Bowen, Frésard et Taillard, 2017)